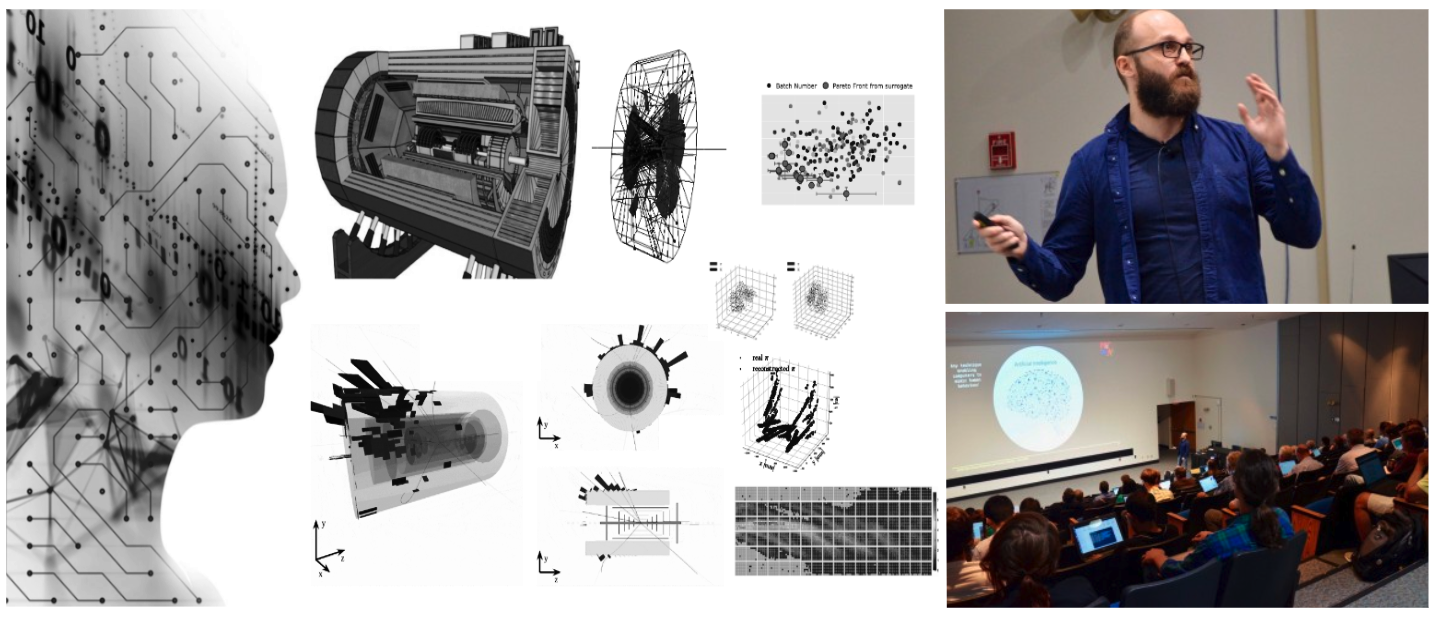
Data Science
Experimental Nuclear Physics
News
Abstract:
We introduce the first method of uncertainty quantification in the domain of Kolmogorov-Arnold Networks, specifically focusing on (Higher Order) Re- LUKANs to enhance computational efficiency given the computational demands of Bayesian methods. The method we propose is general in nature, providing access to both epistemic and aleatoric uncertainties. It is also capable of generalization to other various basis functions. We validate our method through a series of closure tests, including simple one-dimensional functions and application to the domain of (Stochastic) Partial Differential Equations. Referring to the latter, we demon- strate the method’s ability to correctly identify functional dependencies introduced through the inclusion of a stochastic term.
The code supporting this work can be found at https://github.com/wmdataphys/Bayesian-HR-KAN.
Link to the paper:
https://arxiv.org/pdf/2410.01687
This work is the result of a collaboration with Prof. A. Nwala and his team at Data Science, W&M. We utilized our network ELUQuant, originally developed for event-level uncertainty quantification in DIS, for bot detection with uncertainty quantification
Link to the paper: https://arxiv.org/pdf/2407.13929
Abstract:
Social bots remain a major vector for spreading disinformation on social media and a menace to the public. Despite the progress made in developing multiple sophisticated social bot detection algorithms and tools, bot detection remains a challenging, unsolved problem that is fraught with uncertainty due to the heterogeneity of bot behaviors, training data, and detection algorithms. Detection models often disagree on whether to label the same account as bot or human-controlled. However, they do not provide any measure of uncertainty to indicate how much we should trust their results. We propose to address both bot detection and the quantification of uncertainty at the account level — a novel feature of this research. This dual focus is crucial as it allows us to leverage additional information related to the quantified uncertainty of each prediction, thereby enhancing decision-making and improving the reliability of bot classifications. Specifically, our approach facilitates targeted interventions for bots when predictions are made with high confidence and suggests caution (e.g., gathering more data) when predictions are uncertain
AI-assisted Design Bootcamp: it has been really nice to host this bootcamp at W&M, and wonderful interaction with all the attendees. Below a link to the Git Book of this experience, and a picture with the participants
https://aid2e.github.io/boot-camp-2024/intro.html
ArXiv link: https://arxiv.org/pdf/2407.07376
Imaging Cherenkov detectors are crucial for particle identification (PID) in nuclear and particle physics experiments. Fast reconstruction algorithms are essential for near real-time alignment, calibration, data quality control, and efficient analysis. At the future Electron-Ion Collider (EIC), the ePIC detector will feature a dual Ring Imaging Cherenkov (dual-RICH) detector in the hadron direction, a
Detector of Internally Reflected Cherenkov (DIRC) in the barrel, and a proximity focus RICH in the electron direction. This paper focuses on the DIRC detector, which presents complex hit patterns and is also used for PID of pions and kaons in the GlueX experiment at JLab. We present Deep(er)RICH, an extension of the seminal DeepRICH work, offering improved and faster PID compared to traditional methods and, for the first time, fast and accurate simulation. This advancement addresses a major bottleneck in Cherenkov detector simulations involving photon tracking through complex optical elements. Our results leverage advancements in Vision Transformers, specifically hierarchical Swin Transformer and normalizing flows. These methods enable direct learning from real data and the reconstruction of complex topologies. We conclude by discussing the implications and future extensions of this work, which can offer capabilities for PID for multiple cutting- edge experiments like the future EIC.
Dr. Karthik Suresh, a current postdoctoral researcher in Data Science at William & Mary has been awarded the prestigious JSA Ph.D. Thesis Prize for his outstanding doctoral thesis at Jefferson Lab. Dr. Suresh completed his Ph.D. at the University of Regina, where he was co-supervised by Professor Zisis Papandreou and W&M Assistant Professor of Data Science, Cristiano Fanelli. He then transitioned to William & Mary to collaborate with Dr. Fanelli on a grant-funded project focused on AI-assisted Detector Design for the Electron Ion Collider.
M. Diefenthaler, C. Fanelli, L. O. Gerlach, W. Guan, T. Horn, A. Jentsch, M. Lin, K. Nagai, H. Nayak, C. Pecar, K. Suresh, A. Vossen, T. Wang, T. Wenaus (AID (2) E collaboration)
Cristiano Fanelli, James Giroux, Patrick Moran, Hemalata Nayak, Karthik Suresh, Eric Walter
https://arxiv.org/pdf/2404.05752.pdf [2404.05752v1 physics.data-an]
Information on the corresponding hackathon event can be found at https://eic.ai/hackathons
K. Suresh, N. Kackar, L. Schleck, C. Fanelli, arXiv:2403.15729v1 [cs.CL] https://arxiv.org/pdf/2403.15729.pdf
The app can be accessed through https://rags4eic-ai4eic.streamlit.app/
Link to 2023 Hackathon: Physics Event Classification Using Large Language Models (organized by C. Fanelli, J. Giroux, P. Moran, K. Suresh)